- M.A. Lill, Multi-dimensional QSAR in drug discovery. Drug Discov. Today 12(23), 1013–1017 (2007)CrossRefGoogle Scholar
- Hugo Kubinyi, QSAR and 3D QSAR in drug design Part 1: methodology. Drug Discov. Today 2(11), 457–467 (1997)CrossRefGoogle Scholar
- D. Qi-Shi, R.-B. Huang, K.-C. Chou, Recent advances in QSAR and their applications in predicting the activities of chemical molecules, peptides and proteins for drug design. Curr. Protein Pept. Sci. 9(3), 248–259 (2008)CrossRefGoogle Scholar
- C. Hansch, D. Hoekman, A. Leo, D. Weininger, C.D. Selassie et al., Chem-bioinformatics: comparative QSAR at the interface between chemistry and biology. Chem. Rev. 102(3), 783–812 (2002)CrossRefGoogle Scholar
- C. Hansch, A. Leo, D. Hoekman, Albert Leo, Exploring QSAR, vol. 631 (American Chemical Society, Washington, DC, 1995)Google Scholar
- R. Gómez-Bombarelli, D. Duvenaud, J. Hernández-Lobato, J. Aguilera-Iparraguirre, T.D. Hirzel, R.P. Adams, A. Aspuru-Guzik, Automatic chemical design using a data-driven continuous representation of molecules. arXiv:1610.02415 (2016)
- R.L. Camacho-Mendoza, E. Gutierrez-Moreno, E. Guzman-Percastegui, E. Aquino-Torres, J. Cruz-Borbolla, J.A. Rodriguez-Avila et al., Density functional theory and electrochemical studies: structure–efficiency relationship on corrosion inhibition. J. Chem. Inf. Model. 55(11), 2391–2402 (2015)CrossRefGoogle Scholar
- L. Li, X. Zhang, S. Gong, Hongxia Zhao, Yang Bai, Qianshu Li, Lin Ji, The discussion of descriptors for the QSAR model and molecular dynamics simulation of benzimidazole derivatives as corrosion inhibitors. Corros. Sci. 99, 76–88 (2015)CrossRefGoogle Scholar
- M. Karelson, V.S. Lobanov, A.R. Katritzky, Quantum-chemical descriptors in QSAR/QSPR studies. Chem. Rev. 96(3), 1027–1044 (1996)CrossRefGoogle Scholar
- Z. Zhang, N. Tian, L. Wu, L. Zhang, Inhibition of the corrosion of carbon steel in HCL solution by methionine and its derivatives. Corros. Sci. 98, 438–449 (2015)CrossRefGoogle Scholar
- C. Gnerre, M. Catto, F. Leonetti, P. Weber, P.-A. Carrupt, C. Altomare et al., Inhibition of monoamine oxidases by functionalized coumarin derivatives: biological activities, QSARs, and 3D-QSARs. J. Med. Chem. 43(25), 4747–4758 (2000)CrossRefGoogle Scholar
- G. Schüürmann, QSAR analysis of the acute fish toxicity of organic phosphorothionates using theoretically derived molecular descriptors. Environ. Toxicol. Chem. 9(4), 417–428 (1990)CrossRefGoogle Scholar
- Ramon Carbó-Dorca, Stochastic transformation of quantum similarity matrices and their use in quantum QSAR (QQSAR) models. Int. J. Quantum Chem. 79(3), 163–177 (2000)3.0.CO%3B2-0'>CrossRefGoogle Scholar
- M. Rupp, A. Tkatchenko, K.-R. Müller, O.A. Von Lilienfeld, Fast and accurate modeling of molecular atomization energies with machine learning. Phys. Rev. Lett. 108(5), 058301 (2012)Google Scholar
- K. Hansen, G. Montavon, F. Biegler, S. Fazli, M. Rupp, M. Scheffler et al., Assessment and validation of machine learning methods for predicting molecular atomization energies. J. Chem. Theory Comput. 9(8), 3404–3419 (2013)CrossRefGoogle Scholar
- R. Ramakrishnan, O.A. von Lilienfeld, Machine learning, quantum mechanics, and chemical compound space. arXiv:1510.07512 (2015)
- E. Gutiérrez, Development of a predictive model for corrosion inhibition of carbon steel by imidazole and benzimidazole derivatives. Corros. Sci. 108, 23–35 (2016)CrossRefGoogle Scholar
- P.D Lyne, Structure-based virtual screening: an overview. Drug Discov. Today 7(20), 1047–1055 (2002)CrossRefGoogle Scholar
- G.E. Dahl, N. Jaitly, R. Salakhutdinov, Multi-task neural networks for QSAR predictions. arXiv:1406.1231 (2014)
- J. Ma, R.P. Sheridan, A. Liaw, G.E. Dahl, V. Svetnik, Deep neural nets as a method for quantitative structure–activity relationships. J. Chem. Inf. Model. 55(2), 263–274 (2015)CrossRefGoogle Scholar
- T. Unterthiner, A. Mayr, M. Steijaert, J.K. Wegner, H. Ceulemans, S. Hochreiter, Deep learning as an opportunity in virtual screeningGoogle Scholar
- G. Montavon, K. Hansen, S. Fazli, M. Rupp, F. Biegler, A. Ziehe et al., Learning invariant representations of molecules for atomization energy prediction, in Advances in Neural Information Processing Systems (2012), pp. 440–448Google Scholar
- K. Hansen, F. Biegler, R. Ramakrishnan, W. Pronobis, O.A Von Lilienfeld, K.-R. Müller et al., Machine learning predictions of molecular properties: accurate many-body potentials and nonlocality in chemical space. J. Phys. Chem. Lett. 6(12), 2326 (2015)CrossRefGoogle Scholar
- M. Hirn, N. Poilvert, S. Mallat, Quantum energy regression using scattering transforms. arXiv:1502.02077 (2015)
- K.T. Schütt, F. Arbabzadah, S. Chmiela, K.R. Müller, A. Tkatchenko. Quantum-chemical insights from deep tensor neural networks. Nat. Commun. 8 (2017)CrossRefGoogle Scholar
- A. Lusci, G. Pollastri, P. Baldi, Deep architectures and deep learning in chemoinformatics: the prediction of aqueous solubility for drug-like molecules. J. Chem. Inf. Model. 53(7), 1563 (2013)CrossRefGoogle Scholar
- Predicting activities without computing descriptors: graph machines for QSAR §Google Scholar
- C.R. Collins, G.J. Gordon, O.A. von Lilienfeld, D.J. Yaron, Constant size molecular descriptors for use with machine learning. arXiv:1701.0664 (2017)
- Y. LeCun, Y. Bengio, Geoffrey Hinton, Deep learning. Nature 521(7553), 436–444 (2015)CrossRefGoogle Scholar
- D. Ciregan, U. Meier, J. Schmidhuber, Multi-column deep neural networks for image classification, in 2012 IEEE Conference on Computer Vision and Pattern Recognition (CVPR) (IEEE, 2012), pp. 3642–3649Google Scholar
- L. Deng, J. Li, J.-T. Huang, K. Yao, D. Yu, F. Seide et al., Recent advances in deep learning for speech research at microsoft, in 2013 IEEE International Conference on Acoustics, Speech and Signal Processing (IEEE, 2013), pp. 8604–8608Google Scholar
- R. Collobert and J. Weston, A unified architecture for natural language processing: deep neural networks with multitask learning, in Proceedings of the 25th International Conference on Machine Learning (ACM, 2008), pp. 160–167Google Scholar
- D.P. Kingma, M. Welling, Auto-encoding variational Bayes. arXiv:1312.6114 (2013)
- R. Ramakrishnan, P.O. Dral, M. Rupp, O.A. Von Lilienfeld, Quantum chemistry structures and properties of 134 kilo molecules. Sci. Data 1 (2014)Google Scholar
- L. Ruddigkeit, R. Van Deursen, L.C. Blum, J.-L. Reymond, Enumeration of 166 billion organic small molecules in the chemical universe database GDB-17. J. Chem. Inf. Model. 52(11), 2864–2875 (2012)CrossRefGoogle Scholar
- O.G. Mekenyan, G.T. Ankley, G.D. Veith, D.J. Call, QSARs for photoinduced toxicity of aromatic compounds. SAR QSAR Environ. Res. 4(2–3), 139–145 (1995)CrossRefGoogle Scholar
- F. Chollet, Keras. https://github.com/fchollet/keras (2015)
- M. Abadi, A. Agarwal, P. Barham, E. Brevdo, Z. Chen, C. Citro et al., TensorFlow: large-scale machine learning on heterogeneous systems. Software available from www.tensorflow.org (2015)
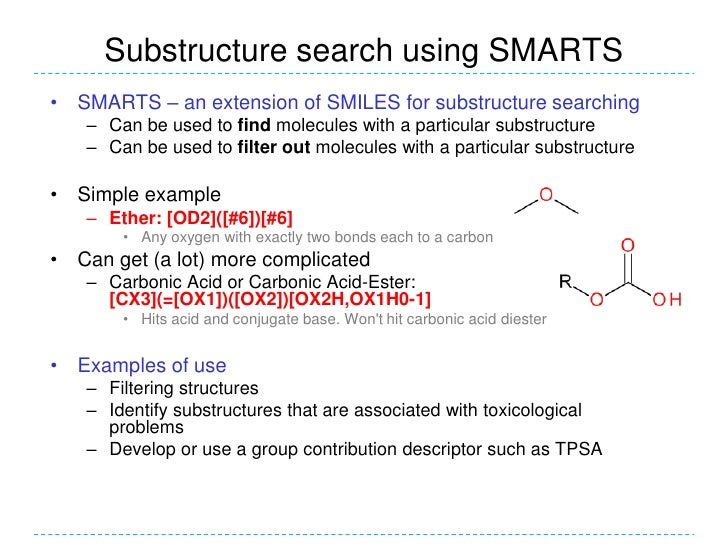
The Biochemical Algorithms Library (BALL) provides an object-oriented C library for structural bioinformatics, and its capabilities include molecular mechanics, support for reading and writing a variety of file formats, protein–ligand scoring, docking, and QSAR modeling. The Chemistry Development Kit (CDK) is a cheminformatics toolkit written in Java. Xii An Introduction to Chemoinformatics One characteristic of chemoinformatics is that the methods must generally be applicable to large numbers of molecules; this has been one of the principles that we have used when deciding what to include in this book. Our emphasis is on the computer manipulation of two- and three-dimensional chemical.
Molecular Descriptors for Chemoinformatics (2nd ed.). By Roberto Todeschini and Viviana Consonni.
Handbook Of Molecular Descriptors
Log in with Open Athens, Shibboleth, or your institutional credentials.
If you have previously obtained access with your personal account, Please log in.
